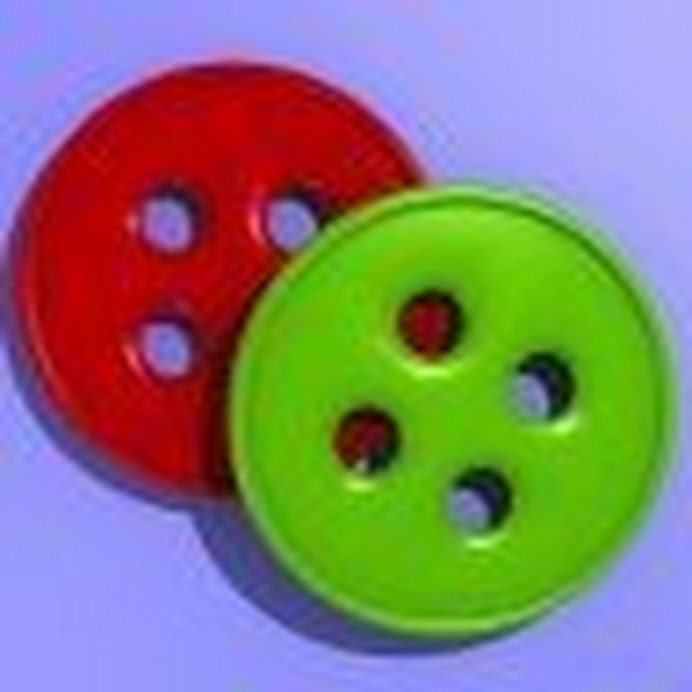
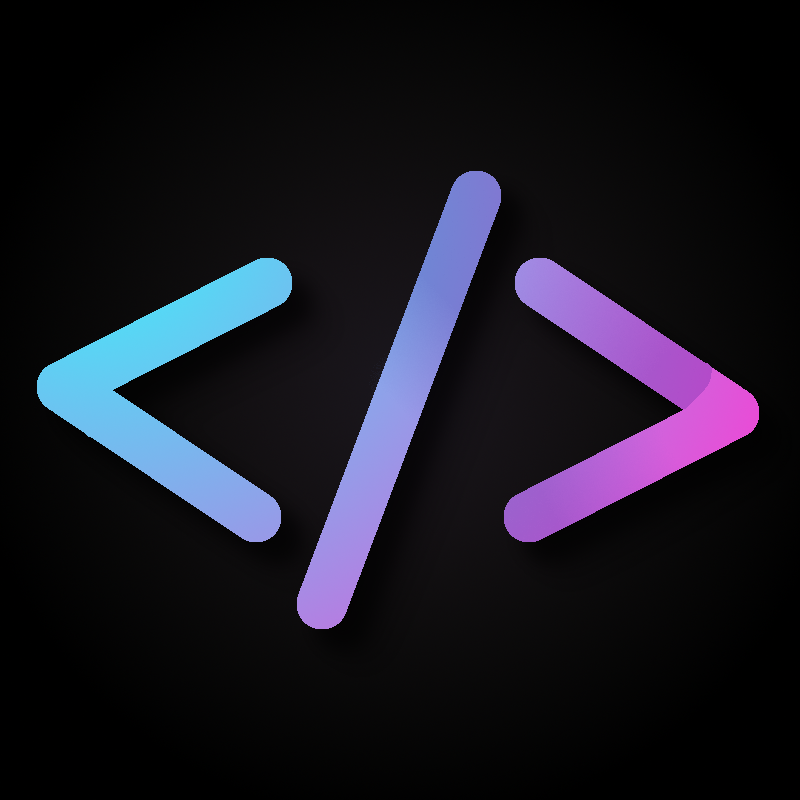
Giving bombs to Israel without condition is a… 6 point story I think
Giving bombs to Israel without condition is a… 6 point story I think
Our bug is their status quo.
Cognitive dissonance.
For a lot of people, either they accept “this trillion dollar corporation that controls all my computers, and the programming languages I use, and my code editor, is evil”. Or they accept “this trillion dollar company does lots of good things for me and is good”.
One is easier to accept than the other.
At first I though it said “Communism is the key to efficiency in a software engineering organization”; at first I thought it said something new
Every commit lists one or more parents, possibly several parents, like 8 parents. These commits thus form a graph structure.
Branches and labels are just references to commits in this graph structure; they are commit alias, just a name that references a specific commit. Branches and tags are the same, except by convention the CLI will move branches when you commit to a branch, but tags are not moved by the CLI.
(Commits may have many names, they have their commit ID, and they may also be named by a branch or tag. Commit IDs are hashes of the contents of the commit. This ensures, cryptographically, that a commit and it’s ID can never change.)
Git never deletes a commit that is less than 90 days old. If you commit something, rest assured your work is in there somewhere, it’s just that no mortal being may be able to find it. Deleting a branch removes a reference to a commit, but the commits in the branch are still there. The GUI tools usually hide commits that are not part of a branch, but you can see them using “reflog” related commands.
Command line is a GUI, change my mind
The CEOs face the day he realizes all it takes to automate his company is a personal computer: 😃
The CEOs face the day after he realizes all it takes to automate his company is a personal computer: 🫠
Can’t people just hold it for 80 years until they die?
People were staring at you because the sound of piss entering the toilet was loud? Did they not know that’s how pissing works?
There are (roughly) two types of projects:
Projects that have coding standards that are documented. If you want to contribute to one of these projects then read the coding documentation and follow it. People will help you write code that fits the standards.
Projects that don’t have coding standards. When you look at such a project you’ll see endless layers of shitty hacks that mostly work, sometimes. Add your own shitty hack to the pile and as long as everything still mostly works, you’re good.
GPT4 did teach me. I say this as the one who learned, whatever that’s worth.
The “trees” for an LLM are their neural networks and word vectors. The forest is a word prediction algorithm. There is no higher level to what they do.
At what level do LLMs teach? Something was teaching me linear algebra and I thought it was the GPT4. When GPT4 was able to recognize a valid mathematical proof that was previously unknown to it, what level was it operating at?
As a programmer I can confirm that LLMs definitely have loops. Look at the code, look at the algorithms, you will see the loops. The “core loop” in the LLM algorithm is “read the context, produce the next work, read the context, produce the next word”.
The core loop in animals is “receive stimulus using senses, move muscles, receive stimulus using senses, move muscles”. That’s all humans do, that’s all animals do.
I think there’s a possibility that humans are simply very advance machines. Look at the debate over whether humans have free will, it’s an interesting question and the important take away is that we still have a lot to learn about our brains and physics. I don’t want to get into that though.
You’ve ignored my main complaint. I said that you treat LLMs and humans at different levels of abstraction:
It’s not fair to say that LLMs simply predict the next word and humans have feelings and reason.
It would be fair though, to say that LLMs simply predict the next word and humans simply bounce electric-chemical signals between neurons and move muscles.
I don’t think that way about people or LLMs though. I think people have feeling and reason, and I think LLMs reason too. LLMs aren’t the same as people and aren’t as good though. But LLMs are good enough to say that they can “reason” in my experience[0].
[0]: I formed this opinion when learning linear algebra from GPT4. It was quite a good teacher. The textbook I’m using made a mistake that GPT4 caught. I encountered a proof that GPT4 wasn’t aware of, and GPT4 wouldn’t agree with me that C(A) = C(AA^T) until I explained the proof, and then GPT4 could finally reason for itself and see for itself that C(A) = C(AA^T). As an experiment, I started a new GPT4 session and repeated the experiment using a faulty proof, but I wasn’t able to convince GPT4 with a faulty proof, it was able to reason through the math concepts well enough to recognize when a mathematical proof was faulty and could not be convinced by a faulty proof. I tried this experiment 4 or 5 times. To be clear, what happened here is that GPT4 was able to learn a near math concept in one shot (within a single context window), but only if accompanied by a proper mathematical proof, and was smart enough to recognize faulty proofs as being faulty. To me, that rises to the level of “reason”.
You apply a reductionist view to LLMs that you do not apply to humans.
LLMs receive words and produce the next word. Humans receive stimulus from their senses and produce muscle movements.
LLMs are in their infancy, but I’m not convinced their “core loop”, so to speak, is any more basic than our own.
In the world of text: text in -> word out
In the physical word: sense stimulation in -> muscle movement out
There’s nothing more to it than that, right?
Well, actually there is more to it than that, we have to look at these things on a higher level. If we believe that humans are more than sense stimulation and muscle movements, then we should also be willing to believe that LLMs are more than just a loop producing one word at a time. We need to assess both at the same level of abstraction.
Somehow the law ignores the giant flashing “Buy!” button but is super concerned about the fine print in 6pt font nobody reads.
First they would need to outright ban practices where you rent your license for an unspecified time instead of owning it.
Why?
People were able to rent games in the past. What happened then that was so bad?
That’s no normal gadget…
I agree. 100 lines of code may be 3x better than 300 lines of code, but 1 line of code isn’t 3x better than 3 lines of code.
Yes. I learned this from Haskell. I like Haskell, but it has a lot of very granular functions.
Earlier comment said that breaking up 1 function into 3 improves readability? Well, if you really want readability then break it up into 30 functions using Haskell. Your single function with 25 lines will become 30 functions, so readable (/s).
In truth, there’s a balance between the two. Breaking things up into function does have advantages, but, as you say, it makes it more likely that you’ll have to jump around a lot to understand a single process.
There’s also websites hosted in countries that don’t care about US law. We can access those even without a VPN, for now…