
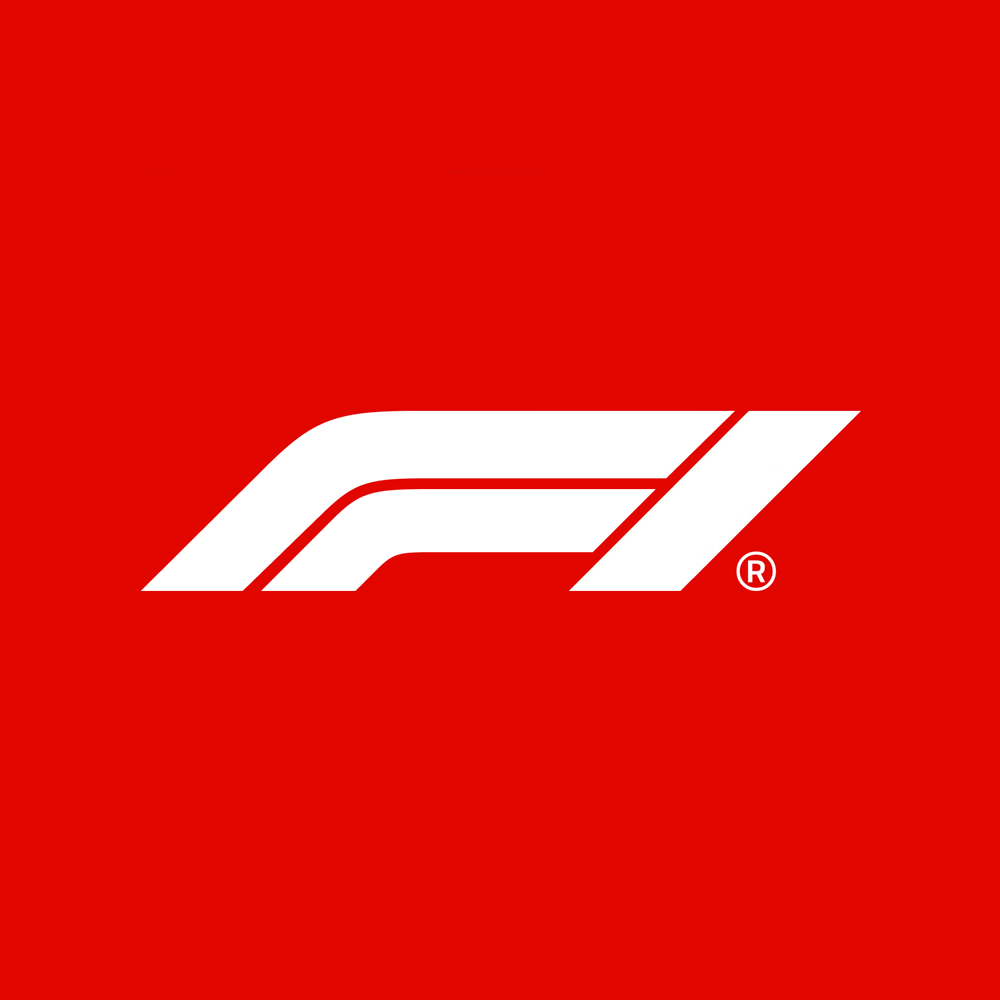
I’ve eaten on about that before, but decades ago when food was cheaper. Nothing is satisfying, you are hungry all the time, constantly craving some nutrition you no longer even know how to acquire or what it is, but it’s absent from everything you eat.
Peanut butter and bread was too expensive. Peanut butter was a treat. Bread from bakery surplus cost two to three times as much as rice. For your example, at $400 a year you’re looking at $8 a week. If a jar of peanut butter is $3 and has 4800 kCal in it and bread is $1 a loaf and has 24 60kCal slices in it, then a jar of peanut butter and 5 loaves of bread a week only gets you 12000 kCal a week, which isn’t enough for a moderately active adult. And you’re going to be missing out on all sorts of nutrition.
At the time the best things to buy were eggs, beans, rice, and processed dry foods. Then you buy things that make eating them bearable and are also cheap in combination: whole or powdered milk to eat cereals, raw sugar, fat to cook into things, very cheap meats, cheese when it was cheap, and processed frozen foods that are similar in price to their constituents, which at the time were common because they are a way of storing food from a production season to sell in an off season. Then you get a few things to try to stave off cravings, like some long-term storage plastic-packed cuts of meat, or canned vegetables, or concentrated frozen fruit. At a low budget a can of food represents everything you get to eat for a day, or more. Fresh vegetables or fruit were completely unobtainable unless there’s a local surplus.
Now the structure of food markets is different and everything is priced based only on demand and not on supply, so frozen processed foods that were available then due to the product being made to take surplus or trimmings and then store them are now priced based on demand for the product. The only things that have stayed similar are the prices for eggs (usually), the cheapest meats (sometimes), staples (usually), and canned foods which are priced based on the cost of transportation and are still routinely too high for such a low budget.
about:config is a weird thing to lock behind ditching Firefox and downloading a different browser